As the COVID-19 virus became a pandemic in March 2020, the amount of (time-stamped written) data, such as news/newspaper reports, scientific articles, social media posts (e.g. blogs and twitter), surveys and other information about the virus and its symptoms, prevention, management and transmission became massively available. Such data contained both valid and reliable information, and relevant facts from trusted sources and also rumors, conspiracy theories and misinformation from unofficial ones.
However, it was not only the amount of (written) data and information about the virus that started to be massively produced, but also the coronavirus’ own vocabulary which has led to a lexical expansion of new word formations, new phrases, and neologisms far beyond what it would have been foreseen. Such content can reveal a wealth of insights into the language and its speakers during that period (e.g., attitudes and concerns) their coping strategies and psychological outcomes, but also provide guidance and information on the development and progression of the disease at the scientific and societal level.
The continuous monitoring of this rather well-defined textual data paves the way for the analysis of the vocabulary, word usage, language constructions and style in these texts using tools from computational corpus linguistics and natural language processing, and it can also support research on COVID-19 from multiple angles. For instance, to study the consequences and effects of political decisions for certain social groups or attitudes towards different groups of people e.g. the elderly or 70+; to gain insights into possible stereotypical uses into the social standing of groups; or to examine the consequences and effects of the crisis and monitor the progression and regression of various events in the news.
In this blog post, we summarize only a few of the very many ways this data can be analyzed, explored and presented and we provide some concrete examples to illustrate how this can be achieved by taking a more language and linguistic perspective described in this blog post. Since at the time of the writing, the COVID-19 situation is fast changing in a complex, and sometimes unpredictable, manner, so the data presented should be seen as a snapshot and results should be interpreted with caution.
The corpus and its composition
The corpus we describe consists of ca 2 500 articles from the time when the first media articles about the new virus started to appear in the Swedish media landscape. The data comes from various Swedish sites, a couple of millions of words, of web-based content from January 2020 to the beginning of January 2021, hereafter the Swedish COVID-19 corpus (sv-COVID-19). The corpus collection is not static and the aim is to enhance and enrich the corpus with regular updates as well as with Twitter and Blog data. Moreover, the content is in plain text format (UTF-8). Due to licensing and privacy restrictions for some of its content, we cannot provide a download. However, we can provide access by making the corpus searchable where the order of individual sentences will be given in a shuffled/randomized order. This will be made available during the beginning of 2021 from the SpråkbankenText’s corpus search interface (Korp; https://spraakbanken.gu.se/korp/#?corpus=sv-covid-19; version-1, updated Feb., 2021).
In all of the examples we provide below, inflected forms and variant spellings of words as well as shortened terms are normalized to their lemma or dictionary form. For example, ‘covid-19’, ‘Covid-19’, ‘COVID-19’, ‘Covid19’, ‘Covid’, ‘2019-nCoV’, ‘cv19’, etc. are converted to the form ‘covid-19’, while ‘coronaviruset’ (the corona virus), ‘corona-viruset’, ‘coronavirusets’ (the corona virus’) etc. are reduced to the dictionary form ‘coronavirus’. This might affect the interpretability for some of the examples in this post; for instance ‘miljard krona’ (billion krona) or ‘social medium’ where almost exclusively would appear in plural form in a text, as ‘miljarder kronor’ (billions of kronor) and ‘sociala medier’. However, it is a common practice in language processing to use the dictionary (a.k.a. lemmatized) forms of words in order to group together the different inflected forms of a word so they can be analysed as a single item. Moreover, the charts below show the frequency of words and word usage since January 2020 where the discussions of the new coronavirus started to appear in the (Swedish) media. The frequencies are often given as absolute frequency values, and sometimes designated as relative frequency ones. Relative frequency is the ratio of the raw frequency of a particular event in a statistical experiment to the total frequency.
Facing a new reality: the coronavirus and its vocabulary
The pandemic vocabulary, words and phrases used by experts and nonprofessionals to describe the crisis soon became frequent and familiar, used and overused in various, partly related discourse contexts. On February 11, 2020, the World Health Organization (WHO) announced an official name for the disease that is causing the 2019 novel coronavirus outbreak. The official new name of this disease became coronavirus disease 2019, abbreviated as COVID-19. Formerly, this disease was referred to in various ways, e.g. as ‘nya coronaviruset’ (new/novel coronavirus), ‘2019-nCoV’ or ‘nCoV-19’. Sometime occurrences were also available in in inaccurate and xenophobic terms, such as ‘Wuhanvirus’ and ‘Kinavirus’ (China virus), two terms frequently used at the beginning of the pandemic. The calendar view in figure 1a illustrates two examples of the raw frequencies of ‘COVID-19’ and ‘coronavirus’ in the Sv-Covid-19 corpus which are (currently) the most frequent content words with over 4500 occurrences for ‘coronavirus’ and over 7000 occurrences for ‘COVID-19’. Note that for the purpose of the graph shown in figures 1a and 1b we do not make any distinction of the two terms, in the sense that many people use both names interchangeably, that is the coronavirus itself that cause the disease commonly known as (COVID-19: Coronavirus Disease 2019) and the disease itself. Each rectangle in figure 1a represents a single calendar day that shows the occurrences of a term, here ‘coronavirus’ (top) and ‘covid-19’ (bottom), each day. White rectangles mean that no occurrences of the term could be found in the Sv-Covid-19. While green rectangles show many occurrences during a specific day according to the legends to the right of the plots.


Figure 1b shows another way to visualize the raw (top panel) and relative (bottom) frequency counts for the two terms. It can be clearly seen that ‘coronavirus’ was overrepresented during the start of the pandemic, at least until the middle of Feb. 2020, when WHO announced the official name of the disease ‘COVID-19’.
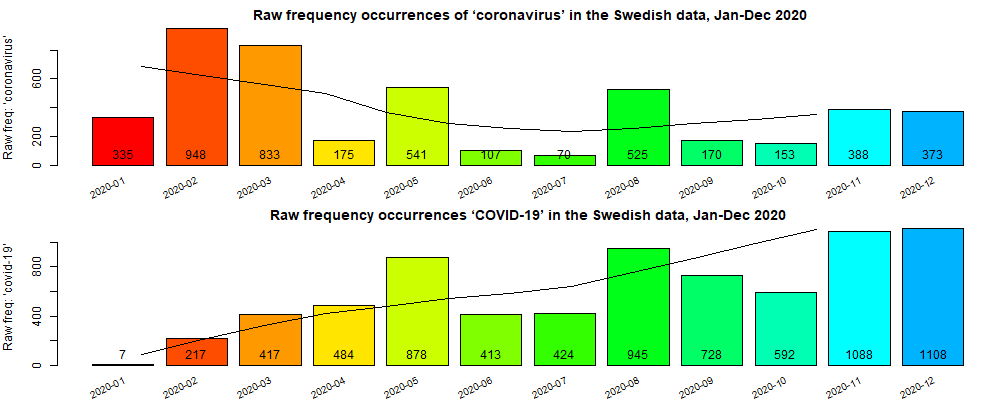
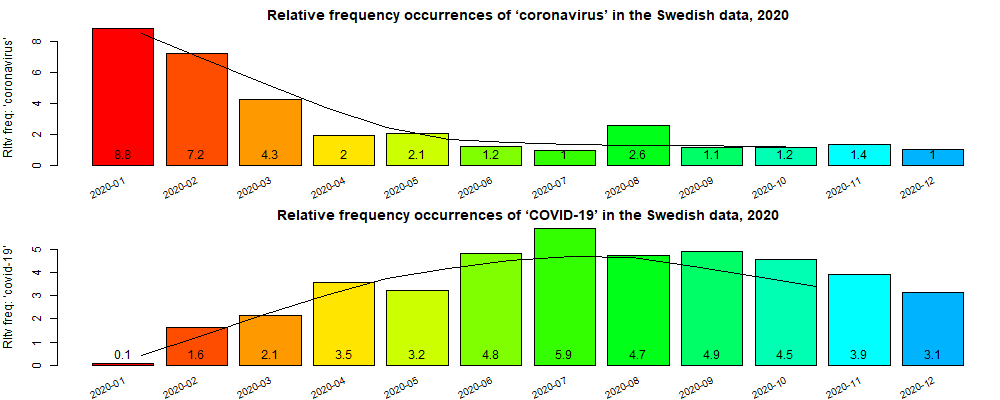
Compounds, compounds and more (new) compounds
Compounds are word forms that consists of a first and a last component. These components can be also compounds, potentially giving rise to very long compound words. Typically, the first component is the modifier and the last component is the head, e.g. in ‘herd immunity’, ‘immunity’ is the head.
Compound and non-compound words, terms and phrases, usually very rare or non-existent in the general discourse during the pre-corona period, such as ‘antikropp’ (antibody) and ‘flockimmunitet’ (herd immunity) became rapidly part of our everyday lives. Even more established words and terms, have rapidly increased in frequency and use compared to the pre-corona period in the general discourse, such as ‘smittspridning’ (spread of infection) and ‘munskydd’ (medical face mask). Others words, usually neologisms and portmanteau words, coined during the beginning of the pandemic period, are continuously produced since then. Some of them dominate the vocabulary during the various development phases of the disease since January 2020 up to now (beginning of January 2021). Such words are for instance ‘covid-19-infektion’ (covid-19 infection) and ‘hemester’ (holiday at home or staycation) and also a long list of high frequency compounds with ‘corona’ or ‘covid’ as modifier, such as ‘coronakris’ (corona crisis), ‘coronastrid’ (corona battle), ‘covid-19-vaccin’ (covid-19 vaccine) and ‘covidsjuka’ (covid sick[ness]).
Figures 2a and 2b show frequency measurements of randomly chosen Swedish compound words over time. Frequencies in single months in the corpus are plotted as line graphs to follow their trends over time, which clearly exhibit different frequency patterns. Note that all calculations have been performed after the data has been converted to lowercase while all inflection forms of the words were converted to their dictionary form or lemma. For comparison reasons, the figures in [square brackets] are raw figures for these compounds taken from the SpråkbankenText’s Korp-system’s newspaper corpora and medical corpora; 50 different corpora (616.36M of 13.43G tokens; https://spraakbanken.gu.se/korp, with a case-insensitive option checked in the extended interface in the 26th of November, 2020).
Figure 2a shows some compounds with ‘corona’ as modifier. These compounds are: ‘coronavaccin’ (corona vaccine [0]), ‘coronastrategi’ (corona strategy [0]), ‘coronavirusutbrott’ (coronavirus outbreak [0]), ‘coronakommission’ (corona commission [0]), ‘coronarestriktion’ (corona restriction [0]) and ‘coronapatient’ (corona patient [0]). Figure 2b shows another small set of compounds with different modifiers; these compounds are: ‘utegångsförbud’ (curfew [2299]), ‘klustersmitta’ (cluster infection [0]), ‘antikroppstest’ (antibody test [25]), ‘iva-plats’ (ICU or intensive care unit bed/place [19]), ‘Hubeiprovins’ (Hubei Province [0]), ‘vaccinsamordnare’ (vaccine coordinator [0]). Note that during counting we also allow a hyphen to appear between the head and the modifier which is common in the data, such as ‘coronapatient’ vs ‘corona-patient’.
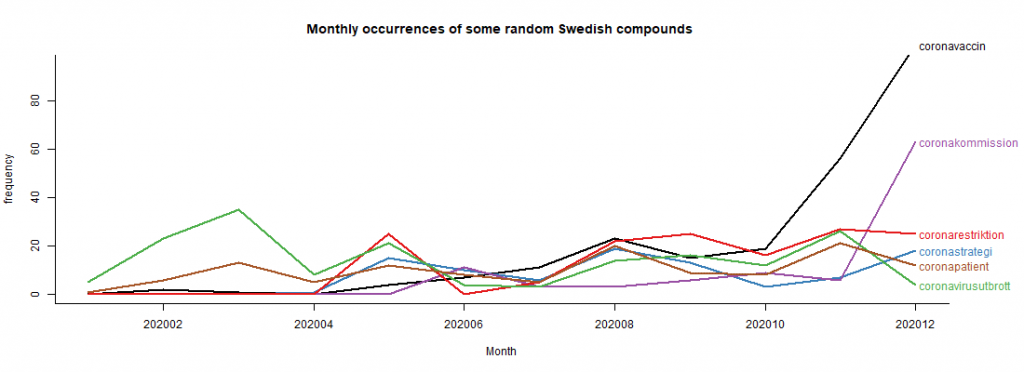
For instance, from the plot in fig. 2a we can see that the frequency mentions of ‘coronavaccin’ and ‘coronakommission’ shows a significant increase during the end of 2020. A similar pattern is for ‘antikroppstest’ (antibody test) that dominates the summer period, with a pick in the middle of the summer and raising again during the end of 2020. Occurrences of ‘Hubeiprovins’ (Hubei province) where high during the first couple of months of the pandemic, rising a bit during the end of 2020, since it is believed that it is exactly one year since the outbreak and several studies suggest that the first case (the “patient zero”) was recorded on December 1, 2019. The ICU-bed occurrences were frequent during March/April and raised again during the second wave of the pandemic in October/December 2020.
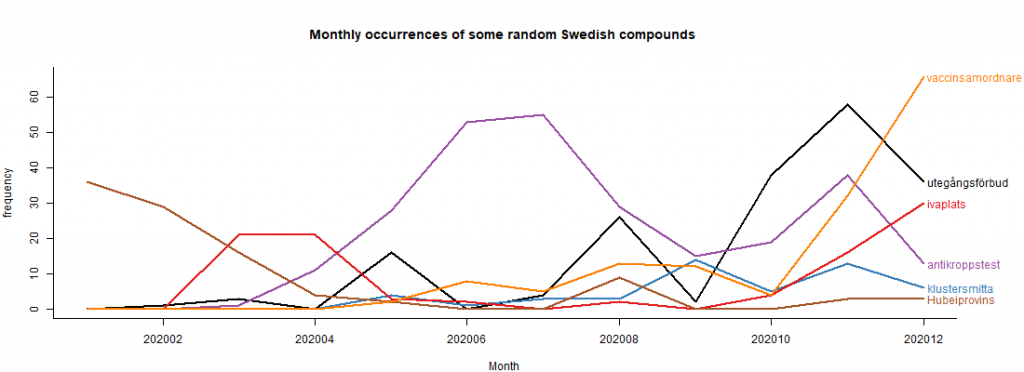
Co-occurrence analysis and collocations
Corpora are invaluable in highlighting the contexts in which a word is used, often indicating particular senses and usages. Collocations are words comprising two or more related words that commonly occur together or near each other in a text, that can be inferred automatically with one or more statistical tests. If two words occur significantly more often as direct neighbors as expected by chance, they can be treated as collocations. The tables and plots below, show the results of a number of collocation measures applied in the Sv-Covid-19 corpus; different methods have their own pros and cons; for instance some methods are more appropriate if the corpus is small in terms of the number of words it includes e.g. a couple of millions of words. Table 1 shows the results for applying the λ (lambda) method used as an association measure for detecting collocations. Table 1 gives the 25 strongest collocations in the sv-COVID-19 based on the λ- and ζ-test (sorted on ζ) and consists of the collocations, their raw counts in the data and the two statistical measures.
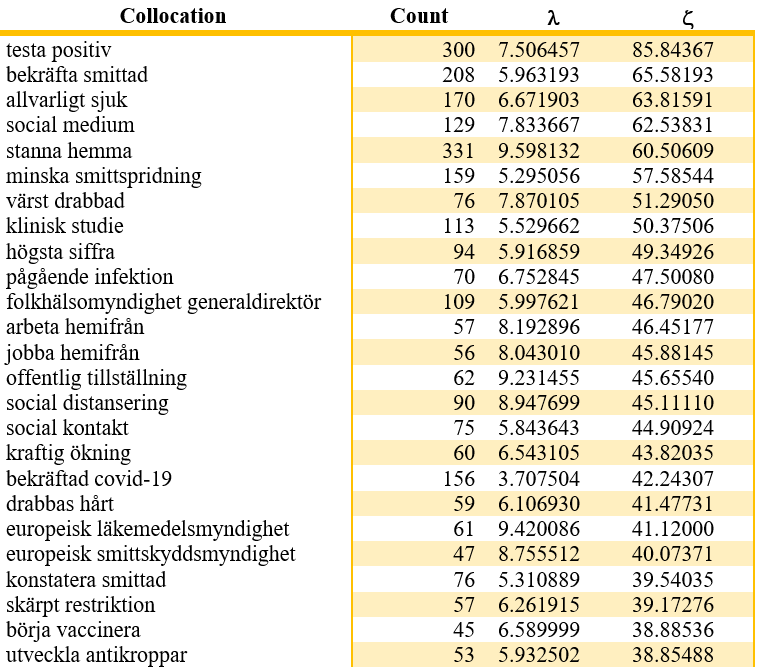
Briefly, co-occurrence analysis methods, such Mutual information (MI), Log-likelihood (LL) or Dice, measures a relationship between two or more random variables that are sampled simultaneously. The Mutual information score measure of how much dependency there is between these variables, or in other words the extent to which observed frequency of co-occurrence differs from what we would expect. The Dice (coefficient) is a basic co-occurrence significance measure that assumes that higher frequency of words means higher significance. LL measures the strength of association between words by comparing the occurrences of words respectively and their occurrences together. A high value simply indicates that there is some correlation between the words. Also, the LL measure is known to be more robust against low expected frequencies and indicates how confidently we can reject the hypothesis that the distributions of the basis and the collocate in a collocation candidate are independent. Note also that in most cases the raw frequency (here ‘Freq-term’) is usually a bad indicator of meaning constitution.
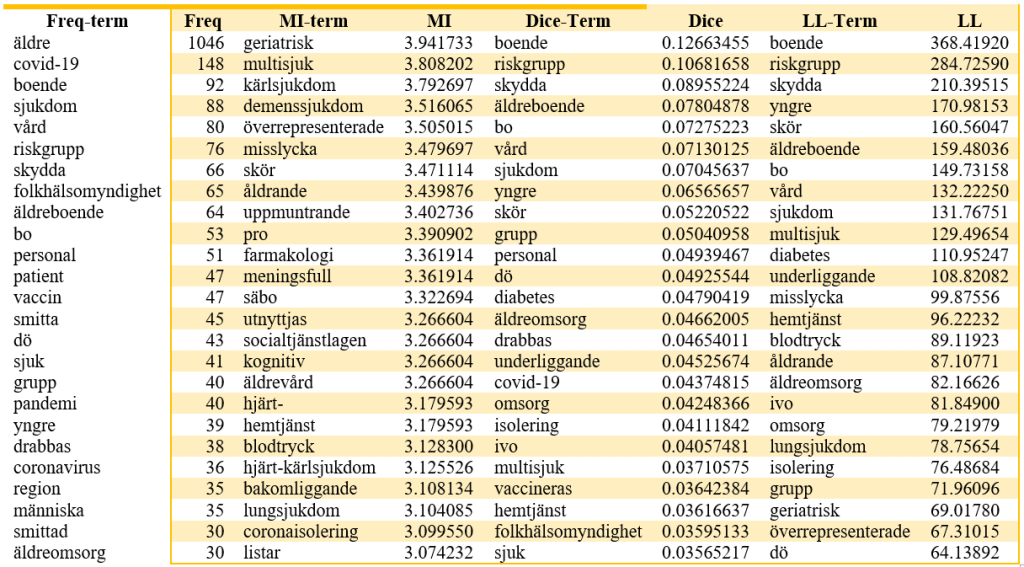
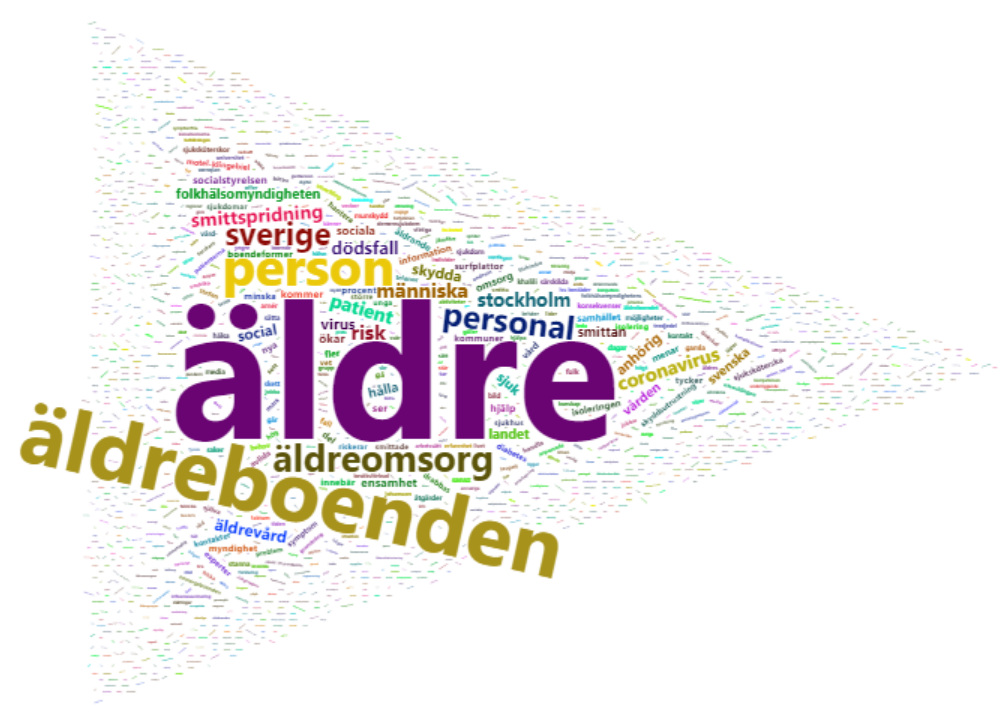
Topic Models
A topic model is a type of statistical model for discovering the latent semantic structures or "topics" (clusters of similar words) that occur in a collection of documents. Topic modeling is a frequently used text-mining tool for discovery of hidden semantic structures in a text body. Intuitively, given that a document is about a particular topic, one would expect particular words to appear in the document more or less frequently: "dog" and "bone" will appear more often in documents about dogs, "cat" and "meow" will appear in documents about cats, and "the" and "is" will appear approximately equally in both. A document typically concerns multiple topics in different proportions; thus, in a document that is 10% about cats and 90% about dogs, there would probably be about 9 times more dog words than cat words (fr Wikipedia). A topic model captures this intuition in a mathematical framework, which allows examining a set of documents and discovering, based on the statistics of the words in each, what the topics might be and what each document's balance of topics is. For the plot in figure 4 we use a method called “Latent Dirichlet Allocation” to visualize the topic proportion over time. Figure 4 shows that topics around “Kina” and “Wuhan” dominate the first two months of 2020 (months 1-2), while “(intensive) care” are the most important topics for March-April (months 3-4) while by the end of the year 2020 (month 12, and even Jan. 2021) there is a domination of topics with "mutations" and "variants".
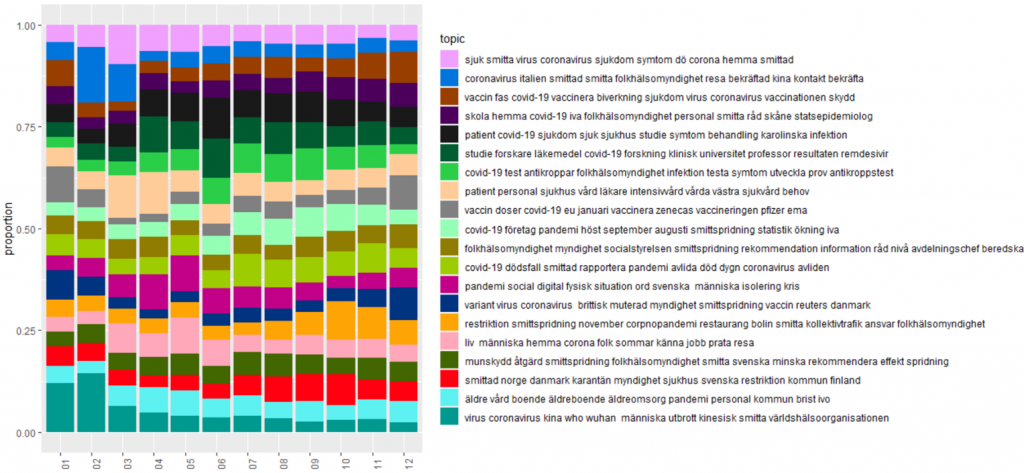